A thorough explanation of business use cases for machine learning
Introduction
A wide range of industries and businesses are using AI and machine learning more and more. Even everyday products like Google’s Alexa and the iPhone’s Siri have incorporated them. AI allows machine learning to learn data, which allows jobs and analyses that would normally take a long time to be finished quickly. The possibilities are unlimited with AI because it can be applied in any way, based on the data it is trained on. This post will outline the application of AI and machine learning in the business world and provide some real-world examples.
What is machine learning? How is it different from AI and deep learning?
To begin with, what precisely is meant by machine learning?
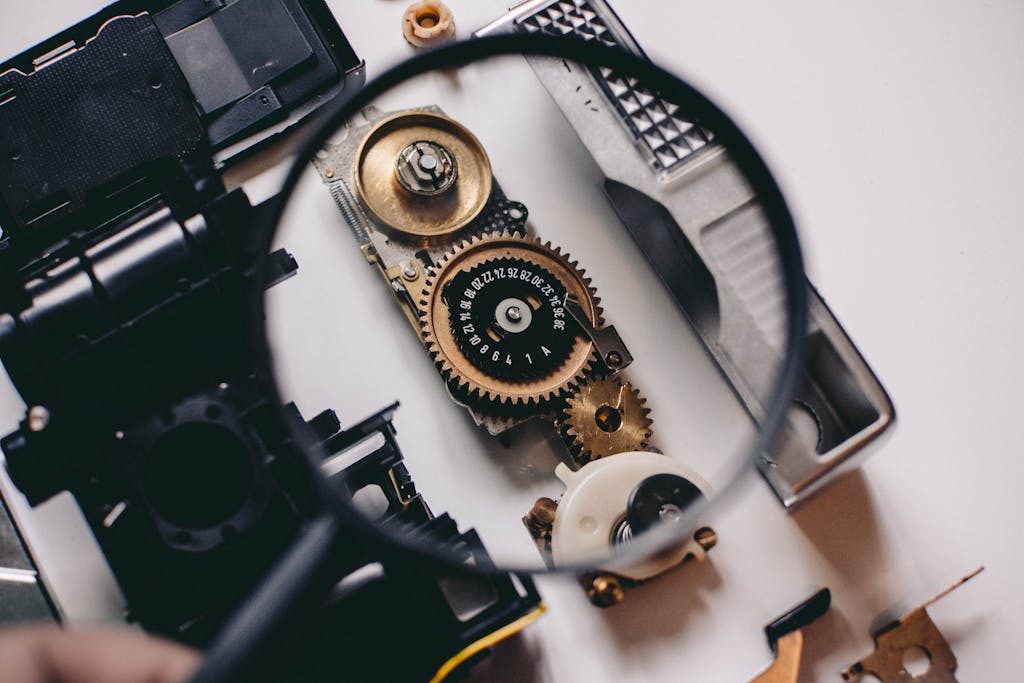
A lot of individuals might not be familiar with the distinctions between machine learning and other words like artificial intelligence (AI) and deep learning.
To begin with, artificial intelligence, or AI, is a machine that mimics human intelligence.
Artificial Intelligence (AI) refers to both the software that can think and analyze, as well as the technology that makes this possible.
Next, machine learning is one of the technologies that supports AI.
This refers to AI reading data, learning from it, and identifying patterns in the learnt data—as the word “learning” implies.
In particular, it is utilized in domains that ask for higher education, such speech and picture recognition through artificial intelligence.
In summary, deep learning is a component of machine learning, which is a subset of artificial intelligence (AI).
Main uses of machine learning
When is artificial intelligence (AI) utilized for machine learning? We
will present three applications: “Image recognition/character recognition,” “Recommendation function,” and “Facial recognition function.”
Face recognition function
Face recognition is the first area in which machine learning is used. This
is a trait that enables AI to recognize faces by mining vast amounts of data for facial feature knowledge.
Face recognition technology makes it possible to increase security by enabling unlocking without a password or key.
In addition, data can be examined to determine the demographics of clients by documenting the number of customers, gender, and age.
Recommendation function
The recommendation function is the second use of machine learning.
AI uses recommendation to suggest appropriate goods and services to each user on a one-to-one basis. Considering the
Using the user’s past purchases and browsing activity, it suggests goods that are related to or bought by people who have similar tastes. It’s
already in use in many different domains, like YouTube’s suggested videos and Amazon’s purchase recommendations.
Character and image recognition
The third use of machine learning is image and character recognition.
We will present a case study of a business that utilized picture and character recognition to cut work time in half as a specific illustration.
One of the responsibilities at a certain manufacturer of industrial parts was entering design drawings into a computer system when an order came in.
The product name, material, and model number are just a few of the details found in design drawings. Approximately 10,000 items of data must be entered each month.
The business was able to cut the significant amount of work time required for reading to input by half by implementing AI.
Now that this labor has been eliminated, time can be saved for tasks that can only be completed by humans.
Machine learning business use cases
Depending on the data that is used to train it, AI can be applied in a multitude of ways.
Examples of machine learning applications in business have been gathered by us.
Store visitor analysis
The analysis of store visitors is one way artificial intelligence is being applied in the retail sector.
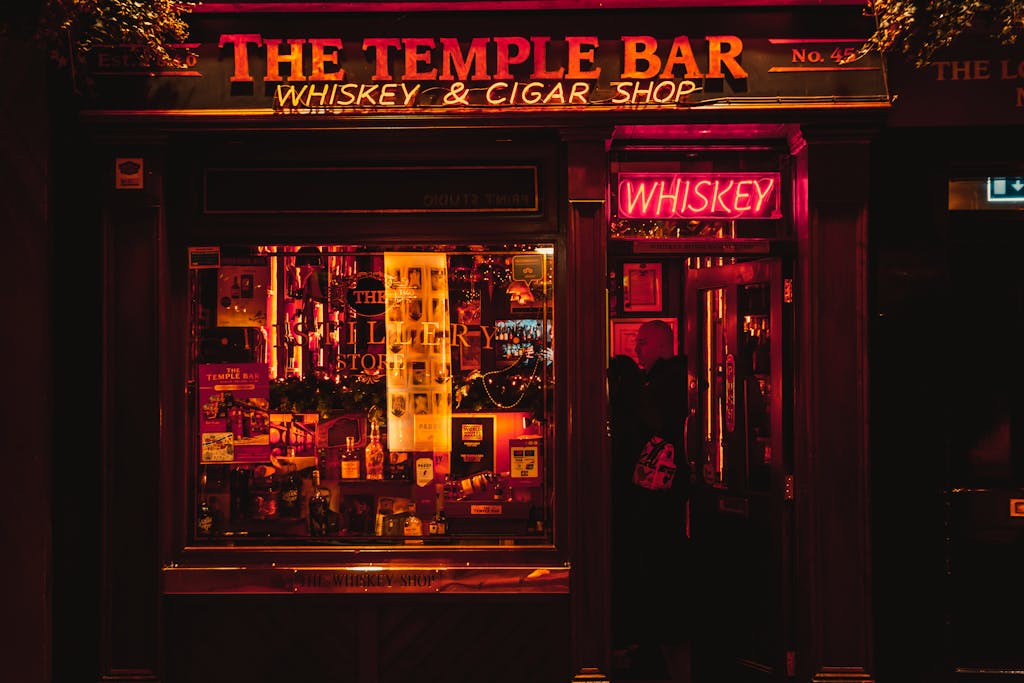
AI is capable of analyzing many types of data, including which route
following their visit to the business, as well as the age and gender of those who made purchases. This was a field that frequently depended on the expertise of specific employees prior to the arrival of AI, but now everyone may objectively examine data.
Additional sales improvements can be achieved by modifying purchase amounts and in-store layouts in accordance with the data analysis.
Farm production forecast
Changes in temperature and precipitation patterns can have a significant impact on the agriculture industry.
Future harvest yields and harvest time can be predicted by applying machine learning to learn from historical harvest data and weather forecasts.
In addition to maintaining stable agricultural crop prices, preventing overproduction improves social issues like decreasing food waste.
It is also possible to estimate the number of workers needed by using the harvest yield, which simplifies the process of forecasting hiring plans.
Apparel demand forecast
Fast fashion serves as an example of how the garment sector is one where trends and fashions cause demand to fluctuate dramatically.
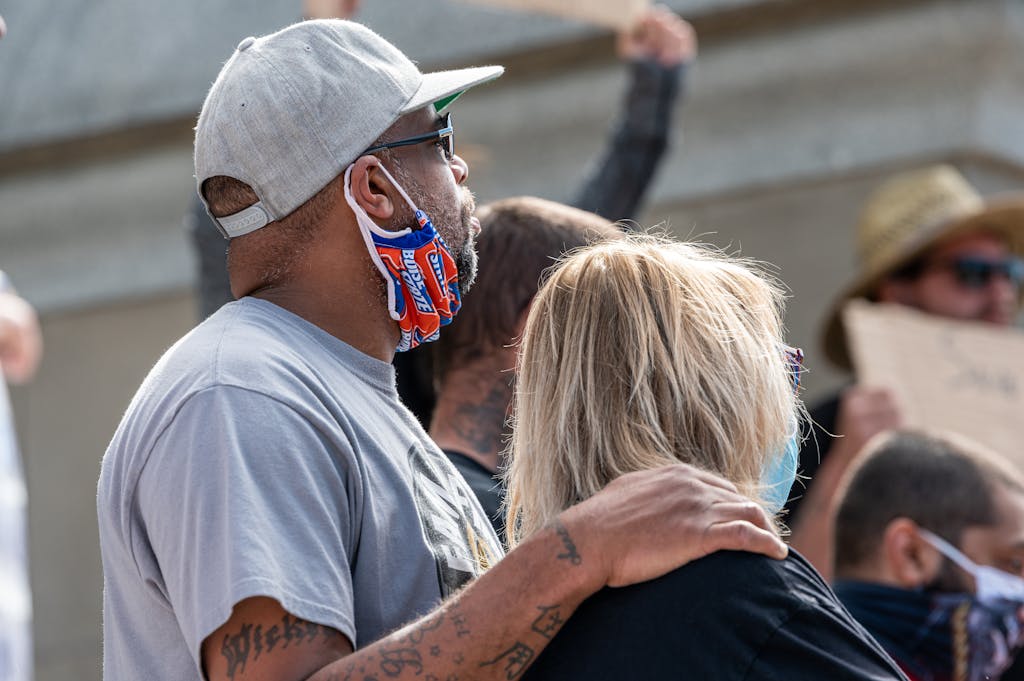
After a few months, popular out-of-stock items become unsaleable, and disposing of excess inventory has turned into a social problem.
It can forecast how much each product will sell during the upcoming few months based on distribution data.
It can also forecast how many things to order by analyzing historical sales data, which helps to avoid having too much inventory.
Response to inquiries via chatbots
You can cut down on the amount of time it takes to answer questions with just the chatbot if you program responses to frequently asked questions.
AI can also be used to allocate queries to the appropriate person to connect them with through the usage of a chatbot.
This is a suggested approach for businesses who struggle with an excessive volume of queries or for services without physical locations.
Call Center Automation
One typical occupation that is always in need of workers is call centers.
Apart from the lack of workers, another issue is that most calls occur on weekends, after work on weekdays, and around lunch, which makes it hard to get through.
Artificial intelligence (AI) with voice recognition capabilities could answer calls automatically in place of people. This
is being used by many businesses because it not only cuts down on the amount of time spent on questions but also helps with workforce shortages and raises customer satisfaction.
Digitization of paper documents
Paper documents can be automatically converted to digital format by integrating AI that can recognize images and characters.
The work involved in deciphering handwritten characters and entering them into the system can be greatly decreased by scanning and digitizing them.
Furthermore, after documents are digitized, there’s no need to keep paper copies, which saves on storage space.
Automatic product identification at cash registers
Because they have numerous locations across the nation, supermarkets and convenience stores are always in need of employees.
It is feasible to check out the products without a cashier by utilizing AI to automatically distinguish the ones being purchased. This
may drastically cut down on the amount of labor needed for cashiering, which not only addresses the labor shortage but also lowers labor expenses.
Sales forecasting
You can estimate future sales by employing AI to leverage machine learning to learn from historical sales data.
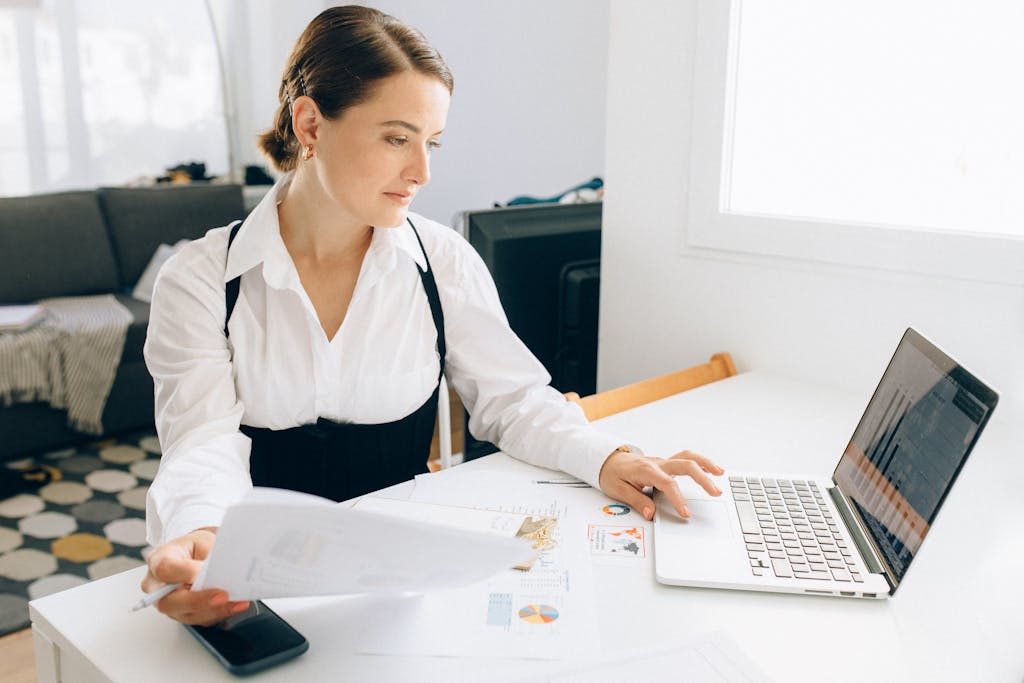
All types of data must be considered in order to estimate sales, but AI is able to do it quickly and with a high degree of accuracy.
You may forecast historical sales benchmarks as well as the amount that advertising and promotional activities will raise sales by.
With this knowledge, you may even plan your response in case your target sales are not met. You
may also calculate the amount of workers needed for each store and estimate the right rates based on sales projections.
Demand and inventory forecasting
Predicting product demand and the right amount of inventory is a very challenging task in the retail sector.
Inaccurate demand projections may cause missed opportunities because of stock shortages or necessitate the sale of surplus inventory at a loss.
Using data from sales performance, inventory levels for individual products, and close competitors, artificial intelligence (AI) may determine the right amount of inventory for each product.
You can minimize costs for less-popular products while increasing the inventory of popular products if you know the ideal levels of inventory for each product.